Quantamental Investing: A Fuzzy Term That Describes An Inevitable Future
Quantamental refers to an investment strategy that combines quantitative approaches using computers, mathematical models, and big data with fundamental methods that analyze individual company cash flows, growth, and risk to generate better risk-adjusted returns.
The term quantamental is a portmanteau combining “quant”itative and fund”amental” investing.
Quantamental investing, the integration of quantitative and fundamental investing methods (think human + machine), is a hot topic on Wall Street. Many seem to agree on the potential but, ironically, few can agree on exactly what “it” is or how firms should best adapt. Beyond the buzzword lies an inevitable future: the integration of quantitative methods into the fundamental investment firm of the future. Here we detail the logic of integrating humans and machines, provide three simple examples and discuss one common mistake.
What Is Quantitative Investing?
Quantitative or systematic trading is essentially computer-driven trading. It involves researcher(s) using math, finance, or trading experience to develop an idea about an exploitable market anomaly such as momentum or price arbitrage. The approach is then validated generally using statistical techniques and either discarded if it doesn’t meet the investment requirements or is turned into a model if it does. Once it’s a model, it’s often programmed on a computer and traded alongside other models automatically; however, some systematic traders place their trades manually.
The logic of quantamental investing is simple. Well-trained human analysts can understand how the future may look different than the past - think of changing industry structures, emerging technologies, shifting company positions or new industry regulations. In these situations, machines focused on historical data are at a disadvantage.
On the other hand, well-developed computer models are unmatched in automating tasks, analyzing how the past may predict the future and identifying anomalies (i.e., automation, prediction, detection). No human can match an algorithm’s ability to vet millions of datapoints in such a manner. Uniquely integrating both presents a logical opportunity.
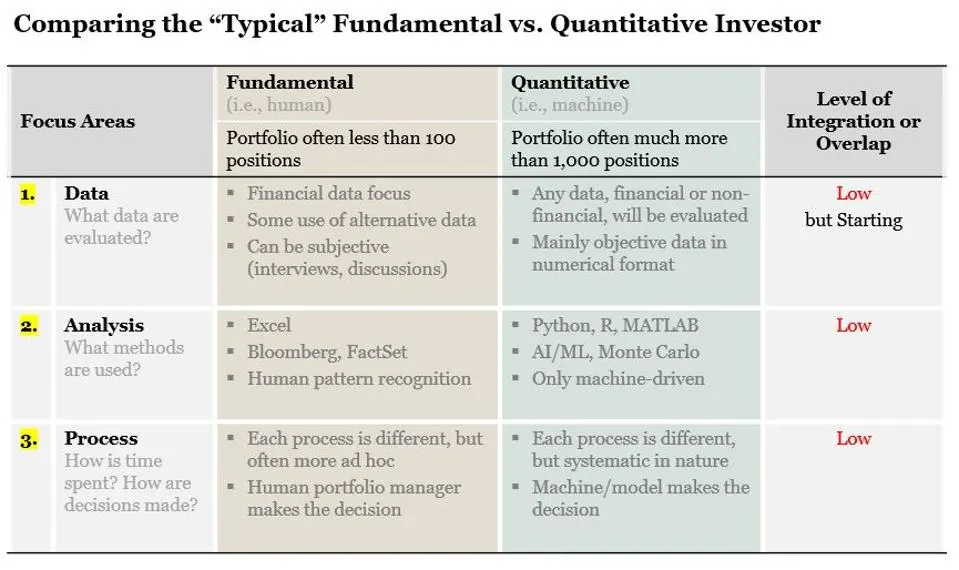
Example 1: Data Edge – Understanding How Businesses are Evolving in Real-Time
Investing is simple, not easy. Simple in the sense that businesses follow clear patterns of growth or decline – and their stock prices tend to follow (see Figure below). While the concept is simple to grasp, it is not always easy to understand how these trends are developing before it is too late. That is where new forms of real-time data can help.
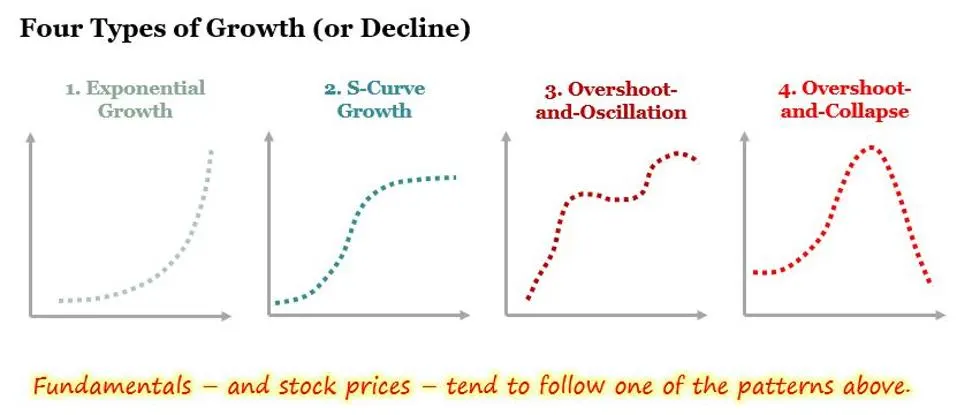
These new forms of data can foreshadow future financial statement results. For example, job postings can help gauge if expansion plans are on track, social media sentiment can be indicative of brand strength and app downloads may be a leading indicator of customer demand. The proliferation of this data is staggering – for example, J.P. Morgan highlighted over 500 alternative data and technology providers (Source: Big Data and AI Strategies: Machine Learning and Alternative Data Approach to Investing, J.P. Morgan, May 2017).
This type of data can be used in a couple of ways. Most traditional fundamental investors will analyze it and simply incorporate it into their existing financial models. For example, if you see app downloads of Netflix are trending down, adjust your Excel model accordingly.
Others may develop more dynamic models that incorporate interdependencies and feedbacks to better understand how growth is transitioning in real-time. For example, timing a transition from exponential to S-curve growth is hard. Stay too long as trends slow and the stock is likely to get crushed. But, if you move off too early you can miss considerable upside. Alternative data sets combined with more robust modeling techniques can evaluate these transitions in real-time (see Figure below).
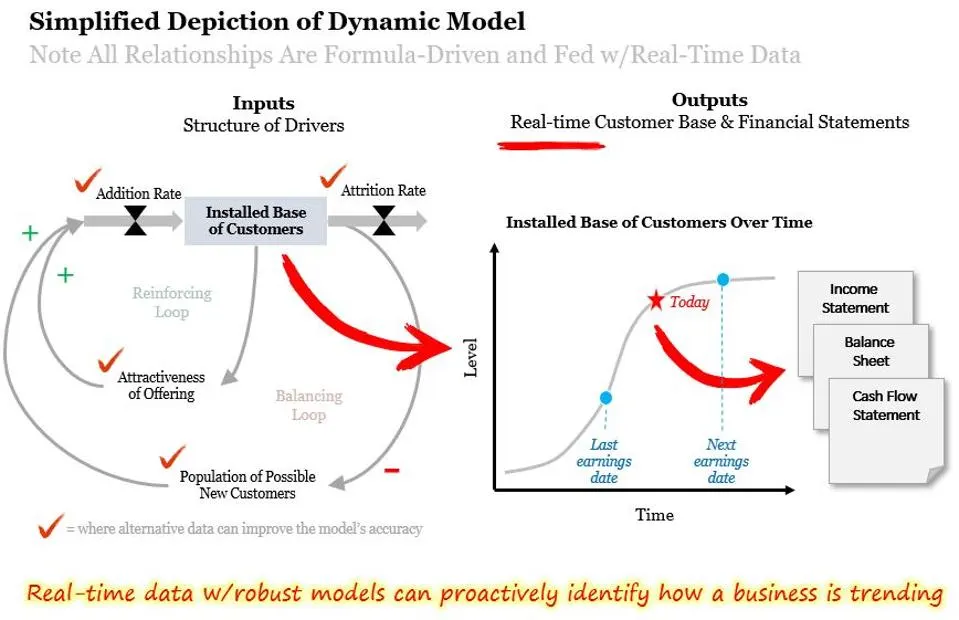
As the proliferation of this type of data grows along with more robust models, the financial statements that come out once per quarter might soon be like reading the newspaper from last month. Interesting for a few tidbits you might have missed, but not informing you of anything material that you did not already know.
Example 2: Analytics Edge – Comprehending Probability Better than the Market
Getting real-time data is one thing, calculating the odds of how it will impact fundamentals and security prices are another. Consider a poker analogy. Alternative data is akin to counting cards; It provides real-time insight into the changing composition of the remaining cards. This is helpful but, to make good decisions, you also need to know other factors such as the value of the pot and the structure of that particular game (i.e., who else is at the table, how they are playing). Then you can more fully understand the odds and make good decisions.
Quantitative methods can monetize the expert analyst’s knowledge in a more robust way. For example, a quantitative model can incorporate the three bullet points above as inputs into a set of simulations. The output from such a model can show that, for example, the company has a 68% chance of beating consensus (see Figure below). This is much more useful than the typical human analyst conclusion of “I think the company will beat consensus.” Do you mean a 51% chance? 85% chance? The implications on sizing and structuring the position (e.g., options) are immensely different in each scenario.
Another way to look at it: integrating quantitative methods can help human analysts discover the asymmetric payoffs that are lurking in their heads but that they are unable to calculate.
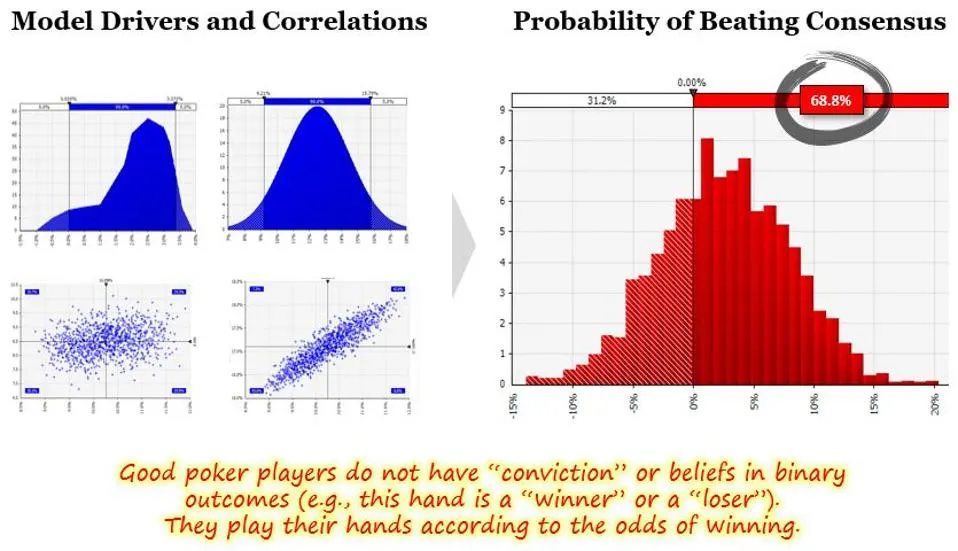
Time is the fundamental analysts most precious, but inefficiently used, resource. Wasted time is like a leaky faucet. At first it can be easy to ignore but, over time, it nearly always leads to problems. An investment process that smartly integrates quantitative techniques can help.
For example, efficiently generating new ideas for the portfolio is always a challenge. At times, analysts can feel like they are bouncing from one random idea to another, wasting valuable time along the way. Integrating well-designed machine learning models (i.e., think pattern detection on steroids) can shortlist high-potential ideas from thousands of possibilities, helping prioritize the human analyst’s research efforts for the next, more in-depth, piece of work
